SNP calling¶
Practical tasks¶
SNP calling with mpileup¶
SNP calling with samtools’ mpileup.
Create a directory named snp_call and download the files.
ngs_user@ngsmachine:~$ cd
ngs_user@ngsmachine:~$ pwd
/home/ngs_user
ngs_user@ngsmachine:~$ mkdir snp_call
ngs_user@ngsmachine:~$ cd snp_call/
Download the reference
and the BAM file
in to this directory.
ngs_user@ngsmachine:~/snp_call$ ls
human_cambridge_reference_mito.fasta mito_yoruba_reads_pe1.sorted.bam
Use Samtools to index the bam file.
ngs_user@ngsmachine:~/snp_call$samtools index mito_yoruba_reads_pe1.sorted.bam
Use mpileup and bcftools to call the SNPs
ngs_user@ngsmachine:~/snp_call$ bcftools view
Usage: bcftools view [options] <in.bcf> [reg]
Options: -c SNP calling
-v output potential variant sites only (force -c)
-g call genotypes at variant sites (force -c)
-b output BCF instead of VCF
(...)
ngs_user@machine:~/snp_call$ samtools mpileup -ugf human_cambridge_reference_mito.fasta mito_yoruba_reads_pe1.sorted.bam | bcftools call -cv - > var.raw.vcf
[mpileup] 1 samples in 1 input files
<mpileup> Set max per-file depth to 8000
[afs] 0:15459.489 1:10.430 2:54.081
ngs_user@machine:~/snp_call$ bcftools view var.raw.vcf | vcfutils.pl varFilter -D100 > var_mito_yoruba_mpileup.flt.vcf
The resulting vcf file contains our SNPs. There are plenty of options to tweak the SNP calling process. To get an idea of the possibilities go to the mpileup page.
Looking at the SNPs using IGV¶
In the IGV we can load the BAM, GFF and the VCF files. In that way we can compare the mapping with the annotation. To do it, open IGV and load the BAM file from the mapping subdirectory. This time you won’t need to import the reference genome, it will be automatically selected, because it was the last reference used. Load the BAM file as you did the last time. Also load the compressed and indexed VCF file in annotation/features/ (you could sort and index the vcf file with an IGV tool). Now you should have two tracks in IGV, one with the mapping and another one with the SNP annotation. We could also load the GFF file with all the annotations, such us SSRs, ORFs, etc. In this case the GFF and VCF tracks would show similar annotation.
Sometimes IGV shows the GFF track collapsed by default, you can expand it by clicking on the right mouse button above the track and selecting expand.
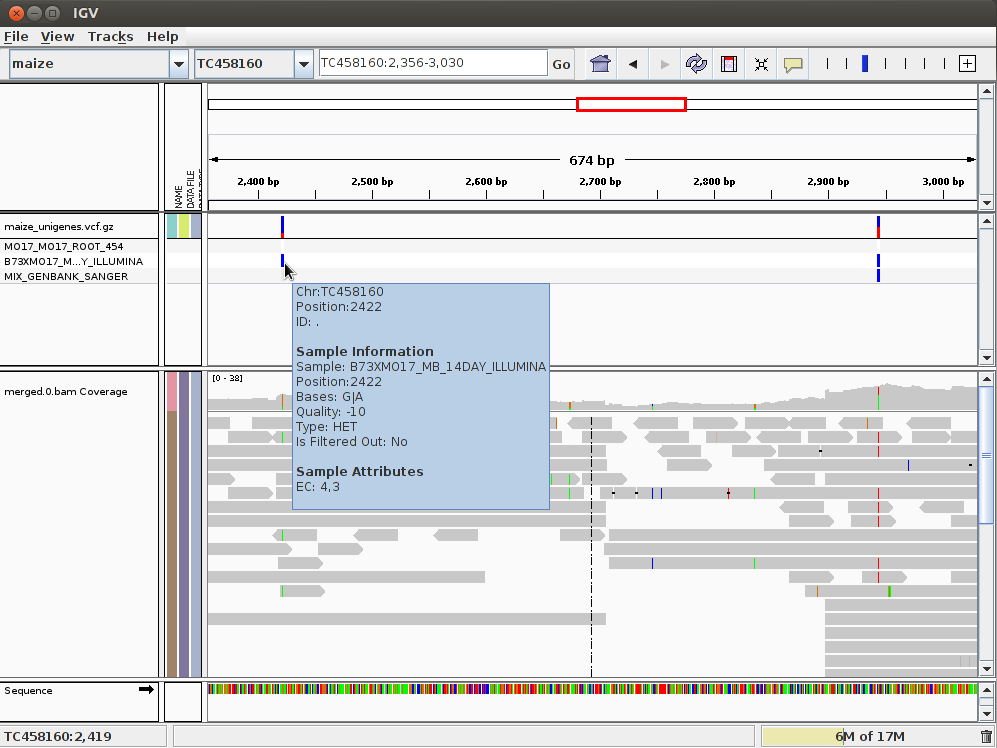
SNP calling with FreeBayes¶
Freebayes is a SNP calling program based on bayesian statistics. It is able to deal with individual and populations or pooled and polyploid samples. FreeBayes is versatil and ajustable, then is necesary to deal with their parameters and options.
ngs_user@ngsmachine:~/snp_call$ freebayes -h
Use FreeBayes to identify SNPs in the previous files.:
ngs_user@ngsmachine:~/snp_call$ freebayes -f human_cambridge_reference_mito.fasta -b mito_yoruba_reads_pe1.sorted.bam -v ./var_mito_yoruba_freebayes.vcf
We have used the FreeBayes with the default configuration and our sample is haploid, run again with this parameter modified.
ngs_user@ngsmachine:~/snp_call$ freebayes -f human_cambridge_reference_mito.fasta -b mito_yoruba_reads_pe1.sorted.bam -p 1 -v ./var_mito_yoruba_freebayes_haplo.vcf
It is posible improve the result with quality paramenters such as:
- -m –min-mapping-quality Q
Exclude alignments from analysis if they have a mapping quality less than Q. default: 30
- -q –min-base-quality Q
Exclude alleles from analysis if their supporting base quality is less than Q. default: 20
- -C –min-alternate-count N
Require at least this count of observations supporting an alternate allele within a single individual in order to evaluate the position. default: 1
Run now limiting to alternate alleles detected in three or more reads.:
ngs_user@ngsmachine:~/snp_call$ freebayes -f human_cambridge_reference_mito.fasta -b mito_yoruba_reads_pe1.sorted.bam -p 1 -C 3 -v ./var_mito_yoruba_freebayes_haplo_reads3.vcf
SNP calling with VarScan¶
The VarsScan is a SNP calling than works with more simple statistics that may be more robust in extreme read depth, pooled samples, and contaminated or impure samples. VarScan employs statistics based on thresholds for read depth, base quality, variant allele frequency, etc.
First is necessary to change the alignement file from BAM to mpileuo format using samtools.
ngs_user@ngsmachine:~/snp_call$ samtools mpileup -f human_cambridge_reference_mito.fasta mito_yoruba_reads_pe1.sorted.bam >mito_yoruba_reads_pe1.mpileup
There are several programs in Varscan.
ngs_user@ngsmachine:~/snp_call$ varscan
mpileup snp is the comand to identify SNPs, but it do not call indels.
ngs_user@ngsmachine:~/snp_call$ varscan mpileup2snp -h
ngs_user@ngsmachine:~/snp_call$ varscan mpileup2snp mito_yoruba_reads_pe1.mpileup -output-vcf 1 >var_mito_yoruba_varscan.vcf
Using IGV, compare the mito_yoruba_haplo_reads3.vcf and mito_yoruba_snps.vcf files, why have not VarScan detected SNPs called by Freebayes?. We have used this option by default.
--strand-filter Ignore variants with >90% support on one strand [1]
Look for SNPs without this strand filter.
varscan mpileup2snp mito_yoruba_reads_pe1.mpileup --strand-filter 0 -output-vcf 1 >var_mito_yoruba_snps_varscan_not_filter_strand.vcf
What did happen with the mileup snps?. Detect the mistake on the command.
SNP calling using calmd¶
Use freebayes to identify snps in these Celegans alignments
against this reference sequence
. Take into account that this bam file has not been processed by samtools calmd. Search for snps with pre calmd processed alignment file and with the post processed file.
ngs_user@ngsmachine:~/snp_call$ samtools index alignments.bam
ngs_user@ngsmachine:~/snp_call$ freebayes -f ref.fasta --min-base-quality 20 alignments.bam |bgzip > alignments.vcf.gz
ngs_user@ngsmachine:~/snp_call$ tabix -p vcf alignments.vcf.gz
Now look for the snvs after procesing the alignment file with calmd
ngs_user@ngsmachine:~/snp_call$ samtools calmd -Arb alignments.bam ref.fasta > alignments.calmd.bam
ngs_user@ngsmachine:~/snp_call$ samtools index alignments.calmd.bam
ngs_user@ngsmachine:~/snp_call$ freebayes -f ref.fasta --min-base-quality 20 alignments.calmd.bam |bgzip > alignments.calmd.vcf.gz
ngs_user@ngsmachine:~/snp_call$ tabix -p vcf alignments.calmd.vcf.gz
Look for the differences using IGV. Take a look at the position 7950020.
If you load the bam files into IGV, can you see differences between them?
We have a VCF file
from a RIL segregant population.
We can use vcftools to filter and to do some statistics.
Filter SNPs with quality minor than 10:
$ vcftools --gzvcf ril.vcf.gz --recode --recode-INFO-all --minQ 10 --stdout | gzip -c > ril_vcf_Q10only.vcf.gz
Filter SNPs with more than 20% of missing data:
$ vcftools --gzvcf ril.vcf.gz --recode --recode-INFO-all --max-missing 0.8 --stdout | gzip -c > ril_vcf_0.8_missing.vcf.gz
Select all SNPs in a determiante region:
$ vcftools --gzvcf ril.vcf.gz --recode --recode-INFO-all --chr CP4_pseudomolecule00 --from-bp 161000 --to-bp 245000 --stdout | gzip -c > ril_vcf_candidate-region.vcf.gz
Calculate the observed heterozygosity of all SNPs:
$ vcftools --gzvcf ril.vcf.gz --het --out ril
Calculate the SNP density:
$ vcftools --gzvcf ril.vcf.gz --SNPdensity 10000 --out ril